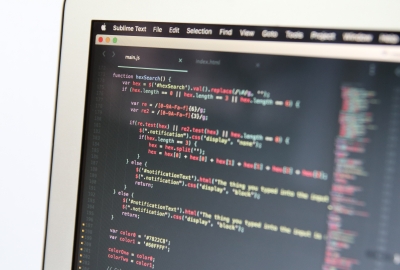
The Methodology and Measurement initiative concentrates on the development, testing, and dissemination of statistical techniques to understand real-world issues. Selected projects include:
- Building and extending methods to explore the sensitivity of inferences of causal effects to changes in structural assumptions, and creating practical guidelines for using sensitivity analyses in applied settings.
- Developing nonparametric Bayesian models at the intersection of machine learning and causal inference, and formulating computational techniques to fit these models.
- Applying modern psychometric methods to improve the reliability and validity of in-class observational measures of teaching, and connecting these improvements to advances in the application of value-added modeling.
Grants and Projects
ThinkCausal: Practical Tools for Understanding and Implementing Causal Inference Methods
Jennifer Hill, Marc Scott, George Perrett, Meryle Weinstein, and Yoav Bergner
Purpose: Causal inference is a necessary tool in education research for answering pressing and ever- evolving questions around policy and practice. However the data typically available to address these questions are often not ideal for causal inference. Most often these data are from observational studies, not controlled or natural experiments, requiring researchers to justify the required assumptions by con- trolling for many covariates. While a large number of (primarily propensity-score based) tools have been developed to estimate average treatment effects in this setting the wide array of choices can leave the applied researcher feeling more confused than supported. Moreover these methods tend to be sensitive to choices with regard to diagnostics with no clear decision points for determinations of critical features such as sufficient balance and overlap. This can inadvertently lead to data dredging or p-hacking practices that are anathema to the advancement of science (Simmons et al. 2011; Gelman and Loken 2014).
At the same time “big data” methods that use automated machine learning algorithms to estimate causal effects are emerging as strong competitors in this arena. These methods take some of the guess- work out of analyses and decrease the opportunity for “p-hacking” (Simmons et al. 2011; Gelman and Loken 2014). Moreover these methods are often better suited for more fine-tuned causal inference tasks such as identifying varying treatment effects and generalizing results from one population to another (Kern et al. 2016; Carnegie et al. 2019a). One such strategy, based on Bayesian Additive Regression Trees (BART; Chipman et al. 2007, 2010), already has a proven track record of superior performance in causal inference settings (Hill 2011; Hill et al. 2011; Hill and Su 2013; Dorie et al. 2016; Wendling et al. 2018). This project will create a user-friendly software package to support researchers in understanding this method and underlying assumptions for causal inference thus leading to higher quality research on causal questions in education research.
Methodological Development: The project team proposes development of a highly-scaffolded multi-purpose causal inference software package, thinkCausal, with the BART predictive algorithm as a foundation. This will allow education researchers from varied backgrounds to access and better understand these powerful and versatile estimation tools. The software will scaffold the researcher through the data analytic process, from uploading data all the way through to graphical and tabular displays of results. It will provide automatic documentation of the analytic choices made. In addition interactive educational components will be interspersed throughout to provide researchers with the opportunity to gain a deeper understanding of how the methods work and their underlying assumptions. Finally the core BART “engine” will be extended to accommodate a wider range of data structures than is typically available in any of the off-the-shelf causal inference methods. The team believes this package will lead to both stronger analyses and more accurate and clear write-ups of results.
Research Design and Methods: The development of this user-friendly software will be embedded within an explicit and iterative design and testing environment. This process will help ensure that we are meeting the needs of applied education researchers. Additionally, we will get feedback on and disseminate the product both locally – through our education research colleagues at NYU Steinhardt – and nationally– through our Advisory Board research network and training sessions at relevant conferences. The performance of the methodological extensions to the underlying BART software in R will be evaluated using simulations that extend the corpus of 7700 datasets created for the 2016 Causal Inference Data Analysis Challenge (three of the current team members were of the organizers of this competition).