
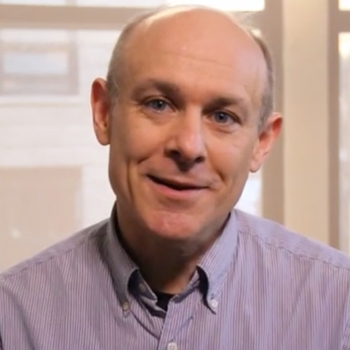
Marc Scott
Co-Department Chair, Professor of Applied Statistics; Co-Director of PRIISM
Applied Statistics, Social Science, and Humanities
PRIISM
Dr. Scott's research involves the development of statistical models for longitudinal data. Using latent variable approaches, he develops new classes of models for such data. He has used them to examine trends in wage inequality, with more recent applications including medical histories and psychological profiles. He also works on models for longitudinal sequence analysis. In the educational setting, such models examine the influence of the entire pathway (e.g., the timing of educational and employment spells and interruptions to these) on an outcome measure such as wages or degree completion. In work on low-wage labor markets, such models seek to find similar structure in the career histories and education of workers to identify more and less successful career ladders. More recent work involves multi-channel and model-based sequence analysis, sensitivity analysis for multilevel models and Bayesian computational methods.
Dr. Scott teaches Multi-Level Models: Growth Curves / Nested Data (APSTA-GE.2040/41/42), Practicum in Statistical Computing (APSTA-GE.2352), Supervised and Unsupervised Machine Learning (APSTA-GE.2011), and Spatial Statistics (APSTA-GE.2015). He serves as co-director with Lisa Stulberg of the Interdepartmental Research Studies (IDRS) program. In Fall 2008, Steinhardt launched a new applied statistics center, PRIISM (Center for Practice and Research at the Intersection of Information, Society, and Methodology), for which he and Jennifer Hill served as co-directors. In 2014, Steinhardt launched a new Masters of Science in Applied Statistics for Social Research (MS-A3SR), for which he and Jennifer Hill served as co-directors.
In Fall 2020, Dr. Scott assumed the role of Chair, Department of Applied Statistics, Social Science, and Humanities. Prof. Jennifer Hill assumed the role of director of the PRIISM Center, and he became deputy director.
Research Interests (with some suggested links):
- Bayesian Models
- Categorical Sequence Analysis
- Causal Inference
- Model-Based Clustering
- Multilevel Models
- Single Subject Designs
Selected Publications
- Marc A. Scott, Jacques-Antoine Gauthier, and Jean-Marie Le Goff. History matters: the statistical modelling of the life course. Quality & Quantity (2023). doi: 10.1007/s11135-023-01648-1
- Joseph Marlo, Marc A. Scott and Sharon L. Weinberg. Who's minding the children: Gender equity in the first two years of the pandemic, International Journal of Social Welfare (2023), 1-20. doi.org/10.1111/ijsw.12622
- Marc A. Scott, Kaushik Mohan and Jacques-Antoine Gauthier. Model-Based Clustering and Analysis of Life History Data. Journal of the Royal Statistical Society: Series A -- Statistics in Society (2020), 1231-1251. Software here.
- Vincent Dorie, Jennifer Hill, Uri Shalit, Marc Scott, and Dan Cervone. Automated versus do- it-yourself methods for causal inference: Lessons learned from a data analysis competition. Statistical Science 34 (2019), 43-68.
- Marc A. Scott, Ronli Diakow, Jennifer L. Hill, Joel A. Middleton. Potential for Bias Inflation with Grouped Data: A Comparison of Estimators and a Sensitivity Analysis Strategy. Observational Studies (2018).
- Marc A. Scott and Matthew Zeidenberg. Order or Chaos? Understanding Career Mobility Using Categorical Clustering and Information Theory. Longitudinal and Life Course Studies vol. 7 (2016), 320-346.
- Joel A. Middleton, Marc A. Scott, Ronli Diakow, Jennifer L. Hill. Bias Amplification and Bias Unmasking. Political Analysis vol. 24 (2016), 307-323.
- Ying Lu, Heng Peng, and Marc Scott. Penalized Fixed Effects Model for Clustered and Longitudinal Data. Proceedings of the 28th International Workshop on Statistical Modelling, (Jean-François Dupuy, Julie Josse, editors), vol. 1(2016), 171-176.
- Ying Lu, Marc Scott, and Preeti Raghavan. Statistical Inference for Single-Case Design: Application to Post-stroke Rehabilitation. Proceedings of the 28th International Workshop on Statistical Modelling, (Jean-François Dupuy, Julie Josse, editors), vol. 1 (2016), 177-182.
- Saul G. Alamilla, Marc A. Scott, and Diane L. Hughes. “The Relationship between Individual and Community-Level Sociocultural and Neighborhood Factors on the Mental Health of Ethnocultural Groups in Two Large U.S. Cities.” Journal of Community Psychology 44 (2016): 51–77.
- Matthew Zeidenberg, Marc Scott and Clive Belfield. `What About the Non-Completers? The Labor Market Returns to Progress in Community College.' Economics of Education Review 49 (2015): 142–56.
- Leoandra Onnie Rogers, Marc A. Scott, Niobe Way. “Racial and gender identity among Black adolescent males: An intersectionality perspective.” Child Development 86 (2015): 407-24.