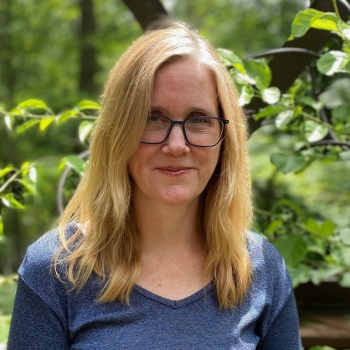
Jennifer Hill
Professor of Applied Statistics; Co-Department Chair; Co-Director of PRIISM
Applied Statistics, Social Science, and Humanities
Jennifer Hill develops and evaluates methods to help answer the types of causal questions that are vital to policy research and scientific development. In particular she focuses on situations in which it is difficult or impossible to perform traditional randomized experiments, or when even seemingly pristine study designs are complicated by missing data or hierarchically structured data. Most recently Hill has been pursuing two intersecting strands of research. The first focuses on Bayesian nonparametric methods that allow for flexible estimation of causal models and are less time-consuming and more precise than competing methods (e.g. propensity score approaches). The second line of work pursues strategies for exploring the impact of violations of typical causal inference assumptions such as ignorability (all confounders measured) and common support (overlap). Hill has published in a variety of leading journals including Journal of the American Statistical Association, Statistical Science, American Political Science Review, American Journal of Public Health, and Developmental Psychology. Hill earned her PhD in Statistics at Harvard University in 2000 and completed a post-doctoral fellowship in Child and Family Policy at Columbia University's School of Social Work in 2002.
Hill is currently the Co-Chair of the Department of Applied Statistics, Social Science, and Humanities (ASH) Department as well as the Co-Director of the Center for Practice and Research at the Intersection of Information, Society, and Methodology (PRIISM). She was the co-founder of the Master's of Science Program in Applied Statistics for Social Science Research (A3SR). The A3SR program has concentration in Data Science for Social Impact. As far as we know this is the first degree granting program in Statistics or Data Science for Social Impact or Social Good in the world. The A3SR program also has a dual degree option with the MPA program at the Wagner School that allows students to earn both degrees in two years.
In 2021, Jennifer Hill was awarded the New York University Distinguished Teaching Award.
To hear more about Hill's perspective on causal inference watch her interview on the SuperDataScience podcast. Out of the 104 episodes recorded in 2022, Hill's was the 2nd most popular.
Hill is excited to share the new software she is developing for machine-learning-based causal inference, thinkCausal, that has an easy-to-use interface and allows users to learn while they use the software. It can be accessed here.
Selected Publications
Check out my recent interview about Causal Inference on the SuperDataScience podcast! You can find it here: www.superdatascience.com/607
- Dorie, Vincent, George Perrett, Jennifer L. Hill, and Benjamin Goodrich (2022) “Stan and BART for Causal Inference: Estimating Heterogeneous Treatment Effects Using the Power of Stan and the Flexibility of Machine Learning,” Entropy, 24(12): 1782.
- Harel, D., Seaman, D., Hill, J., King, E., and D. Burde (2022) “The impact of indirect questioning: Asking about you versus your friends,” International Journal of Social Research Methodology, (accepted)
- Gaebler, J., Cai, W., Basse, G., Shroff, R., Goel, and S. and J. Hill (2022) “A causal framework for observational studies of discrimination,” Statistics and Public Policy, 9:1, 26-48.
- Whipps, M., Yoshikawa, H., Demirci, J., and J. Hill (2022) “Painful, yet beautiful, moments: Pathways through Infant Feeding and Dynamic Conceptions of Breastfeeding Success,” in press at Qualitative Health Research, 32(1): 31-47
- Whipps, M., Yoshikawa, H., Demirci, J., and J. Hill (2021) “Estimating the Impact of In-Hospital Infant Formula Supplementation on Breastfeeding Success,” Breastfeeding Medicine, 16(7): 530-538, https://doi.org/10.1089/bfm.2020.0194
- Benway, N., Hitchcock, E.R., McAllister, T., Feeny, G.T., Hill, J., and J. Preston (2021) “Comparing biofeedback types for children with residual /ɹ/ errors in American English: A single case randomization design,” American Journal of Speech-Language Pathology, 30(4): 1819-1845
- Reinstein, I., Hill, J., Cook, D., Lineberry, M., and M. Pusic (2021) “Multi-level longitudinal learning curve regression models integrated with item difficulty metrics for deliberate practice of visual diagnosis: groundwork for adaptive learning,” Advances in Health Sciences Education https://doi.org/10.1007/s10459-021-10027-0
- Gelman, A. and J. Hill (2020) Regression and Other Stories, Cambridge University Press.
- Hill, J., Linero, A., and J. Murray (2020) “Bayesian Additive Regression Trees: A Review and Look Forward,” Annual Review of Statistics and Its Application, 7: 251-278.
- Dorie, V., Hill, J., Shalit, U., Scott, M. and D. Cervone (2019) “Automated versus do-it-yourself methods for causal inference: Lessons learned from a data analysis competition,” Statistical Science (with commentary and rejoinder), 34(1): 43:68, 94-99.
- Scott, M., Diakow, R., Hill, J. and J. Middleton (2018) “Potential for Bias Inflation with Grouped Data: A Comparison of Estimators and a Sensitivity Analysis Strategy,” Observational Studies, 4: 111-149.
- Kern, H., Stuart, E., Hill, J. and D. Green (2016) “Assessing methods for generalizing experimental impact estimates to target samples,” Journal of Research in Educational Effectiveness, 9(1): 103-127.
- Middleton, J., Scott, M., Diakow, R. and J. Hill (2016) “Bias Amplification and Bias Unmasking” Political Analysis, 24(3): 307-323. (Winner of Society for Political Methodology's Miller Prize for the best article appearing in Political Analysis in 2016)
- Hill, J. and Y. Su (2013) “Assessing lack of common support in causal inference using Bayesian nonparametrics: implications for evaluating the effect of breastfeeding on children's cognitive outcomes,” Annals of Applied Statistics, 7(3): 1386-1420.
- Hill, J. (2011) “Bayesian nonparametric modeling for causal inference,” Journal of Computational and Graphical Statistics, 20(1): 217-240.